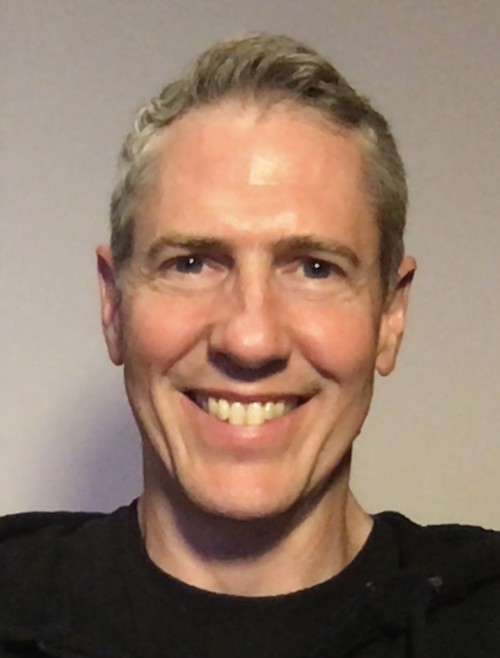
In 2018, Brian Richmond left WeWork, where he founded the people analytics team, to bring his data science skills to a startup in the Bay Area. There, he has leveraged advanced analytics and AI to bring about dramatic improvements in the product and grow engagement rates at Aura Health, where he is Senior Data Scientist, Product Intelligence. Now, as he thinks about the future of the continuously-evolving field of data science, The Data Standard sat down with him to discuss the importance of critical thinking skills and curiosity for students so they enter the workforce with technical knowledge, as well as, as he puts it, an ability to “learn how to learn.” Below is an edited transcript of our conversation.
You left WeWork, where you were running people analytics, to join a small startup in the Bay Area. Talk a little about your role there.
Yeah, WeWork had about 2400 employees when I joined and about 7000 when I left, but always celebrated and supported startups. I loved the entrepreneurial spirit of startups and decided to join one. Aura Health is a very small, early-stage company. We have only four people on site in the US and another four people remote. The CEO created our mobile app and leads our team, his brother is the Chief Product officer, and we have one amazing back-end engineer.
I find that really interesting that a small startup has the awareness to bring on a data scientist, even at the earliest stage.
In fact, I was employee number one. Other than the CEO and Chief Product Officer, I was the first one to join. I think that making the first hire a data scientist is a testament to the data-driven nature of places like the Bay Area and New York City. They realize the importance of making decisions based on data, especially in any kind of tech context. And so, because it’s such a small company, I do wear several hats. I run all the AI that powers our app and learns from data. I also do a lot of basic business intelligence, as well as setting up the data infrastructure so we can actually measure whether something we’re doing has impact or not and whether the impact is positive or not. We’re constantly running experiments, launching several every week. So we’re very agile and can constantly try out different product ideas.
What is Aura Health’s focus and how does data play into it?
Our mission is to help restore the world’s mental health. And so at this time of a global pandemic crisis, we’re actually doing remarkably well because there are so many people out there now struggling with mental health, stressed out, and need help to relax and find peace. Often people come to Aura Health because they have trouble sleeping, but that’s the immediate problem — the root cause of that insomnia is that their mind is spinning and they’re stressed out. Data is at the very core of our product to help people reduce stress, sleep better, and feel more grounded.
What does ‘data science’ mean to you and in your daily work?
It’s still a fairly new term. Data science grew out of the more traditional role of statistician and added more programming and approaches to handle big data. Data science as a field has evolved into being increasingly product oriented. Now with AI, instead of just analyzing what phenomenon is happening, data science involves using AI in products that can learn based on data and make smarter decisions. That’s where I think data science has evolved from a more just analytical role.
At Aura Health, we use AI in our recommendation engine that quickly learns your tastes. So if you try a meditation and don’t like it, then try a different meditation or inspirational life coaching session and like that instead, our AI quickly learns what you like, which coaches you like, what you’re likely to like next time. It’s constantly learning based on what you’re doing in the app. We run a huge neural network model every few hours that updates with fresh data, so that by the next time you log in, Aura now has a different algorithm for you. In a lot of ways, it’s like Spotify. Spotify is brilliant at learning your tastes, and finding music that you might have never heard before. And that you love. This kind of recommendation engine improved Aura’s engagement by about 20%, right away.
I’ve really enjoyed working closely with the product and putting big ideas out there that have improved our engagement and conversion rates. Being at an early-stage startup allows us to make big impacts — not just 2% here, 2% there, but really big impact like improving peoples’ engagement by 10 or 20% or more.
What’s the biggest data challenge you’re having as a company? What are the areas that you are trying to tackle?
The biggest challenge — it’s a pretty common one, and it’s not a sexy one: data quality. Having reliable data that captures all the important signals, doesn’t have too many bugs, and you know what the data actually means. Most businesses have issues where sometimes useful data doesn’t get collected or has bugs. For example, at one point, a bug sometimes recorded a played track hundreds of times. It was throwing our AI out of whack because our AI thought you really liked that track since you played it hundreds of times. That problem wasn’t too hard to fix, because we put more data cleansing checks in place.
Being able to monitor and stay on top of data quality is a big challenge, and we are tackling it in several ways. First, we set up a “Data Dictionary” to record and track our data fields and properties, so everyone works with the same data definitions. Next, we set up many logs and alerts so that we can track data and isolate where bugs happen. And I also have a to-do list to regularly check a bunch of dashboards we’ve created just to see if anything’s really out of whack. It’s not as automated as I’d like, but on a small team we have to prioritize our time and resources.
Data quality is a universal issue.
Yeah. I used to teach statistics at a university. A well-known lesson is that more clean data has a bigger impact than a fancier or better model. It may be fun to try out deep learning or neural networks, because they really do some amazing things that are kind of counterintuitive. But those advanced techniques don’t hold a candle to quality data for many problems. So you can use less sophisticated techniques with really good data and get a better result than a fancy technique without good data. The data quality is really, really key. It’s garbage in garbage out.
You used to teach data science — what are the most important skills that students and professionals should be developing?
The number one thing — the most important thing — is to learn how to learn. Because the tools you’ll use 10 years from now will not be the same as the ones you’re using now. It might even be a different language. So you have to be a student for life. It might sound like a ‘cop out’ answer because it’s also critical to have a strong foundation in basic statistics and statistical theory that leads into the foundations of machine learning. However, that will only take a data scientist so far. It’s important to know how to read up on something, do an online course to learn a new technique, and integrate that with what you already know.
In my opinion, the best way to teach data science is to teach it in a way that forces the student to learn how to get that information without just telling them. If I just tell you exactly how to do something, and you do it, this doesn’t help you out in the real world where you have to actually learn how to solve a problem. Part of the process is not just teaching them to be really smart at one thing, but teaching them how to do something on their own.
Certainly, you can spot the strong foundation in statistics and machine learning in a potential job candidate, but how can you tell if a job candidate has the “learn how to learn” aspect?
One of the best ways to assess is to have them do a project. Give them enough time. A lot of companies will have a candidate come on site and give them an hour to do something. But that’s not a really realistic model of how the job of a data scientist actually works. So if you’re under a super time constraint, and it’s a new brand new problem, and they want you to use new tools, that’s only going to work if you happen to already have done exactly that kind of problem with those tools before. It’s a pretty poor measure of a candidate’s abilities to perform the job.
There are better ways to spot if a job candidate has good foundations in data science and the ability to “learn how to learn.” Uber used a good approach. They gave a take-home challenge that mimicked the kinds of problems Uber was tackling, to be done over the time frame similar to someone working in that role. The candidate then used their own tools to run data analyses and present their data solution and business recommendations. That was a high-yield test, but may be too time-consuming for some candidates. A good alternative is to review a number of the candidate’s previous relevant projects.
How do you see the data science field taking shape over the next few years?
It’s hard to project years out, but one thing is sure: data science, like almost any other field, will become increasingly specialized. You might have people who really specialize in recommendation algorithms or natural language processing. An analogy can be found in medicine, where long ago people had one doctor, and now doctors specialize not just in surgery, or neurosurgery, they can become specialists in pediatric neurosurgery or even more specialized roles.
Another one of the biggest issues of the future, and most difficult to tackle, is the ethics around AI and the human biases in it. Understanding that and being able to mitigate it is critical and we have barely scratched the surface. There’s going to be a struggle between those who use AI for good and those who don’t.