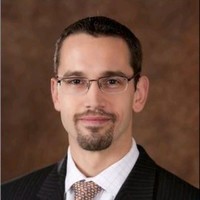
To build or to buy is a common debate within growing businesses. When companies take the “buy” option, their next move is to absorb their acquisitions into the larger business. Andrew Jacobus is health and well being analytics and data science leader at Virgin Pulse, where, among many other things, he has been tasked with just that, integrating data from various businesses into one whole, while keeping up with business model changes and priorities that can conflict with maximizing data’s value, such as agile strategies like minimum viable product that can vie for efficiencies that keep modeling in flux. “There are lots of challenges,” he notes.
Never a dull moment for Jacobus, who took time out of his day to talk with The Data Standard about the role of data science in his company and the field at large.
What do you do at Virgin Pulse?
We’re a health and well-being company, a separately-owned subsidiary of the Richard Branson Virgin family of companies. We provide software to help people get and stay healthy and happy, with a mobile app to reinforce daily habits. I’m part of the Virgin Pulse Institute, which is more of the research and development and thought leadership arm, my team are called the Insights and Data Science team.
We’ve got a spectrum of folks doing work, from data engineering through statistical modeling. We’ve done some AI work, but it’s not really going into application too much. In between, we do research on how effective is our software and what can we do to make it better. How can we leverage insights to strengthen our clients’ approaches to getting the most uptake and the best impact with our products and services. All with the purpose of trying to get people to be healthier and happier, but also to be more productive.
How big is your team, in the context of the company and your area?
We’re 10 people, which is not bad for a company of only 1500. And, you know, in HR, you don’t usually get that kind of leeway. We’re fortunate for our size, and being a data-driven business, work with other people in the business doing data engineering, data management architecture, that sort of work in service to our software platform.
What are some of the big problems that you’re working on right now?
Well, everybody’s focusing on the coronavirus, and we’re trying to get a position on mental health, which is really difficult to do. Because it’s not something that people have been open about in the past, although we’re seeing more and more folks willing to talk about or track or manage their stress. Our focus is: How are we making a difference in helping people manage their mental health right now. As far as subject matter goes, that’s probably top priority. But because it’s still not a high-tech source of data, being mostly self-reported, if at all, It’s a slow burn.
We are also focused on, creating models that help our clients try to predict more accurately what their utilization is going to be over the course of the year: How many people, given how the companies set up what they offer, are going to sign up? How many people are going to continue to engage and earn points in the gamification at certain levels, with varying levels and types of incentives or rewards involved? So some of it is almost basic financial modeling and forecasting. But some of it is predictive modeling, because we use behaviors, a lot of different inputs that aren’t just, run rates and financial standard metrics. And from those models we build tools using applications like R Shiny from the R tool kit.
With those, we then essentially put self-service analytics in the hands of our coworkers. , So we have things that we do for our company internally, and we have things that we do for clients. And then we also try to translate the learning and insights from all of that for conferences, presentations, webinars, blogs. That’s the thought leadership aspect of it.
It’s demand is all over the map, so we’re not tightly focused.
Is there a particular data challenge you’re having as a company?
Oh, no, there are lots of challenges. We started out as one company in my first month on the job in 2016, then we bought two more companies. And then over the course of the next months and years, up through January of this year, we bought five other companies. So there’s a massive integration and standardization challenge, which we’re still addressing. We have also lost a lot of historical data, so we have questions we can’t answer based on what clients used to look like versus what they look like now. Benchmarking is difficult.
Since our architecture and our data infrastructure are meant to support a software product, not analytics, a lot of the data is incomplete. Much of it is based on minimally viable products, which are created for speed and efficiency, not data maximization. Which, in data science, if the data is not great, your models are not as strong as they could be. So we have a big challenge of comprehension and accuracy in our data. And then there’s also the challenge of bringing disparate source systems together and trying to get all under one cloud-based environment.
This is not unique to us. Even in non M&A environments data accuracy and quality are always going to be a problem because the rate of growth in data and its accessibility are insane. To me, that means there aren’t enough people to keep up with it all, so we need to expand on machine learning and create the automated capabilities to process and analyze all of these things with as much data as possible.
How are you addressing this in your work? What tools are you using?
We’re plugging along and trying to collaborate with the owners of the technology in the business to make sure we’re able to try to keep up with just the growth of data that we have within our own shop. We’re not focusing right now on innovating. And a lot of our clients are losing money due to COVID-19 so I think any plans we had for growth and innovation are put on hold for now.
We would love to be able to take advantage of more machine learning-based artificial intelligence-based applications just to help us with our data processing and speed of analytics. Our company wants to use AI for interaction with people using our applications and our services — AI-based smart chat and smart bots, and recommendations that are more real-time based. Those are high priority, because that stuff is sexy, but they’re low priority because of the complexity and because of the quality of our data, {and demands for resources in other areas].
How do you think that data science is going to take shape over the coming years?
I don’t think it’s going to take shape, I think it’s just going to continue to grow and evolve and be unwieldy. It’s going to require more innovation than ever before, and more creative thinking. There are some scripts you can follow, there are some successes that people can learn from others, especially in a time like now, where the money is not necessarily there. The efforts are going to take even more diligence and discipline and focus, and I think it’s easy for companies that have profit in mind to be distracted.
Are you seeing other trends driving this?
For the last 10 years, analytics has been a huge thing for universities to be teaching. There are lots of data science graduates who still need experience to develop common sense, or theoretical assessment. They’re great working with data. They’re great with using different tools and running models. But when it comes to the application of that stuff, it takes time and it takes experience.
So we’re becoming a more digital world. There’s more and more data to work with. There are more and more processes that are losing rigor because of speed and because of agility. And the whole “let’s get things built as quickly as possible and let it evolve without discipline on the evolution.” That’s going to be kind of a vicious cycle for the field.
If innovation slows due to less focus, skills or money, are we just going to see a data pile-up?
That’s a good way of thinking about it. There’s a logjam of data. There’s a logjam of talent, but it’s all in high demand. We’re not training people well. We’re not taking time on the diligence and the discipline in the establishment of good solid practices, the reinforcement of creativity. And frankly, it’s training and development, giving people the space to evolve, that I feel like is underserved. I don’t think it’s not happening, but it’s the pressure to do so coming from the talent. It’s not coming from the companies as much as it used to.
AJ: Feels like there’s an opportunity for closing thoughts, finishing out the article/interview here.
If so, feel free to use some variation on the following, or give me another call to wrap it up.
Any closing thoughts or recommendations?
I realize I am seeing things and speaking to them through a current prism and the challenges in my own environment, but I feel strongly about the future of this field. Smart people find new ways to learn from data and use it in more and more productive manners, and history says great innovations are born out of crisis. I think we’ll never escape the fundamentals, blocking and tackling, if you will, of rigorous acquisition and management of accurate, high quality data, and serving the masses with solid information and insights with a human interpretation. But I would love to believe there will be some fantastic new advancements in universal data sharing, for instance, or new AI applications to put analytics on steroids and solve major real-world problems in real time. The opportunity is definitely there, and it’s fun to be a part of as it evolves.